Cancer neurodegenerative disorders and other diseases have multiple subtypes, each with their different causes, effective treatments and clinical outcomes. Tumor genome sequences provide a rich new source of data for uncovering these subtypes, but they have proven difficult to compare, as two tumors rarely share the same somatic or germline mutations. The Ideker Lab previously introduced the concept and method of network-based stratification (NBS), which integrates tumor genomes with knowledge of hallmark cancer pathways encoded in gene networks [Chuang et al. Mol Sys Biol 2007 and Hofree et al. Nature Methods 2013; first translated to the clinic in Chuang et al. Blood 2012]. This approach allows for stratification of cancer neurodegenerative disorders and other diseases into informative subtypes by clustering together patients with molecular alteration in similar network regions (e.g. distinct mutations in the same hallmark pathway). These network-defined subtypes have turned out to be predictive of clinical outcomes such as patient survival, response to therapy or tumor histology. Thus far the evidence suggests that network biomarkers, which aggregate together mutations in multiple genes, will not just be useful in clinical interpretation of cancer genomes, in many cases they may be necessary.
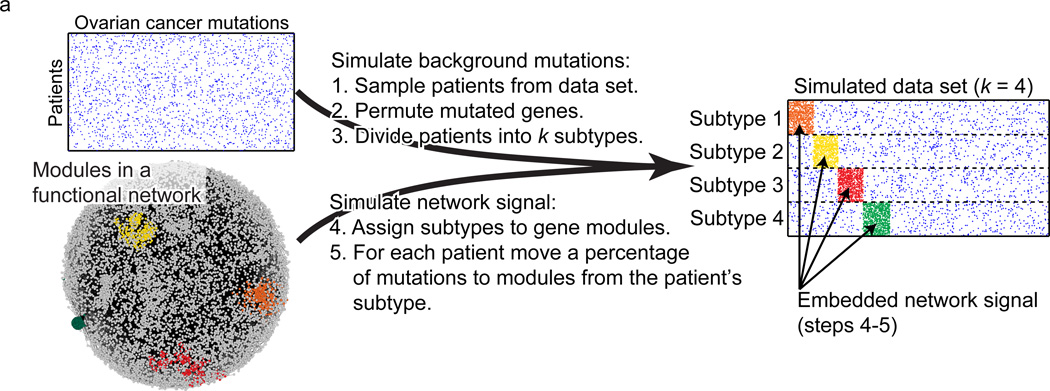
Network-based stratification of tumor mutations. Figure 2a.
[Hofree et al. Nature Methods 2013]
We made substantial progress in translating such network and pathway analysis from an initial proof-of-concept to a robust practice and set of informatic tools for research and clinical use. This research includes work to exhaustively evaluate and rank the publicly available molecular network databases based on their ability to aggregate and interpret the genetic alterations observed in different tumor populations [Huang et al. Cell Systems 2018] as well as a stable open-source implementation of the NBS algorithm [Huang et al. Bioinformatics 2018]. It also includes a supervised variant of the approach [Zhang et al. Bioinformatics 2018] as the original approach was unsupervised, as well as a demonstration that some outside knowledge of cancer cell biology will be required if we are to continue to identify cancer genes, most of which are rarely mutated [Hofree et al., Nature Communications 2016].

Systematic Evaluation of Molecular Networks for Discovery of Disease Genes. Graphical Abstract. [Huang et al. Cell Systems 2018]
We have also extended network analysis of coding mutations to analysis of non-coding mutations. In this recent work we used a very large network of enhancer-gene interactions, originally mapped by the ENCODE project, to analyze the whole-genome sequences of 930 tumors. This analysis identified 193 mutation hotspots in the non-coding genome which are both recurrently mutated in cancer and for which mutation leads to a substantial effect on expression of the downstream target genes. The majority of these hotspots are observed again in a second large cohort, and three have thus far been shown to validate in functional assays [Zhang et al. Nature Genetics 2018]. We have also identified interactions between non-coding germline variants and later somatic events, such as positive selection for somatic mutations in particular tumor suppressors or oncogenes [Carter et al. Cancer Discovery 2017].
These works have also stimulated studies by many other research groups who have further advanced the methods and identified networks underlying different human diseases and stages of development. For an example of others’ recent work using NBS, see Fujimoto et al. Whole-genome mutational landscape and characterization of noncoding and structural mutations in liver cancer [Fujimoto et al. Nature Genetics 2016].
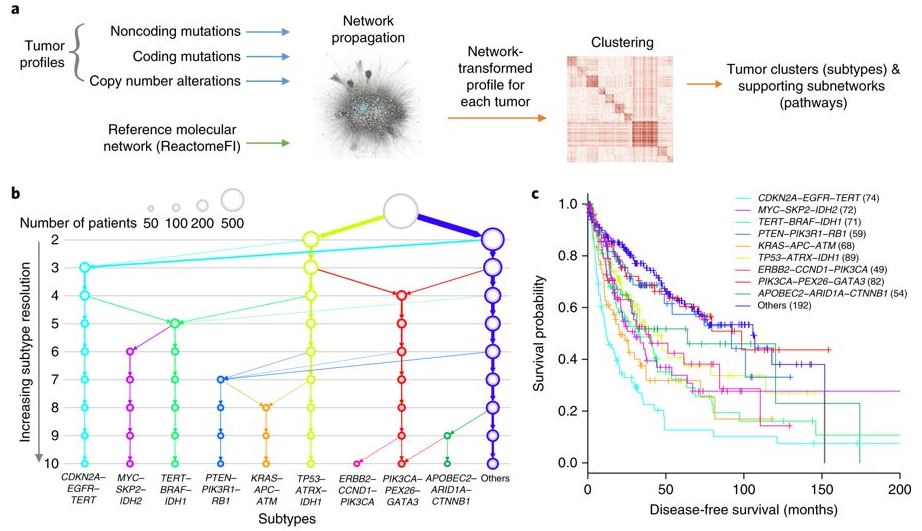
A global transcriptional network connecting noncoding mutations to changes in tumor gene expression. (Fig.5) Identification of molecular networks and associated tumor subtypes incorporating noncoding mutations. [Zhang et al. Nat Gen 2018]
Highlights from the past few years include a study of epistatic interactions among the mutations found in tumor genomes (Van de Haar et al. Cell 2019) as well as a review, with Jonathan Flint, providing guidelines for use of molecular networks in studies of genome-wide association for psychiatric disorders (Flint and Ideker, PLoS Genetics, 2019). Finally, I am co-corresponding author on three papers (together with Nevan Krogan) which comprehensively map the protein networks underlying multiple tumor types and use these maps to identify >300 protein complexes and larger protein assemblies under mutational selection in cancer. This new work includes published comprehensive protein interaction networks for breast cancer (Kim et al. Science 2021) and head-and-neck cancer (Swaney et al., Science 2021). A third paper analyzes these and other network data to identify a large compendium of protein complexes under selective pressure for mutation (Zheng et al. Science 2021). These papers were published as back-to-back articles in the same issue; they were the result of a more than five-year collaboration between my laboratory and that of Nevan Krogan at UCSF, with significant contributions from the laboratories of Silvio Gutkind (Chair of UCSD Pharmacology), Stephanie Fraley (UCSD Bioengineering) and others.
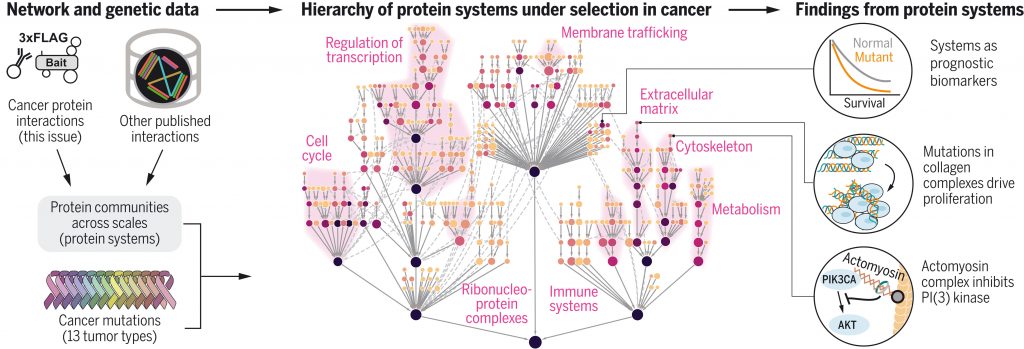
Fan Zheng* & Mark Kelly*, et al. Interpretation of cancer mutations using a multiscale map of protein systems. Science. 2021 Oct 1. *Equal Contribution [PDF] [PubMed]
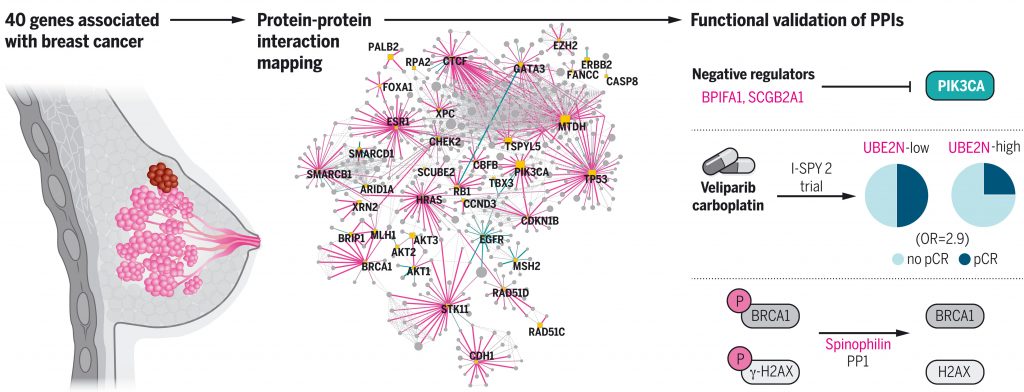
Kim M, et al. A protein interaction landscape of breast cancer. Science. 2021 Oct 1. [PDF] [PubMed]
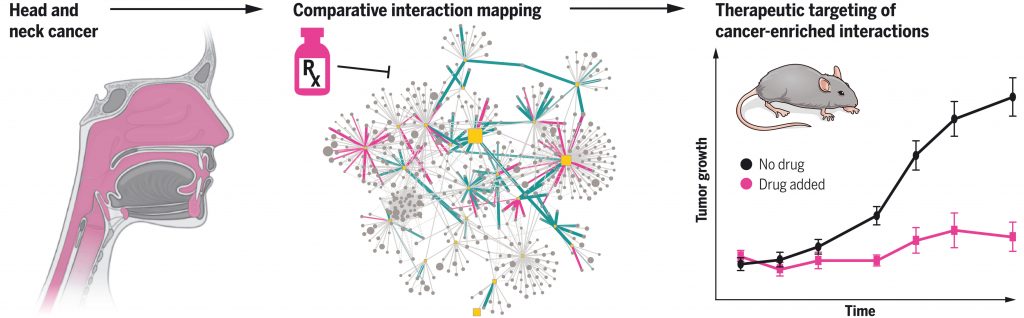
Swaney DL, et al. A protein network map of head and neck cancer reveals PIK3CA mutant drug sensitivity. Science. 2021 Oct 1. [PDF] [PubMed]
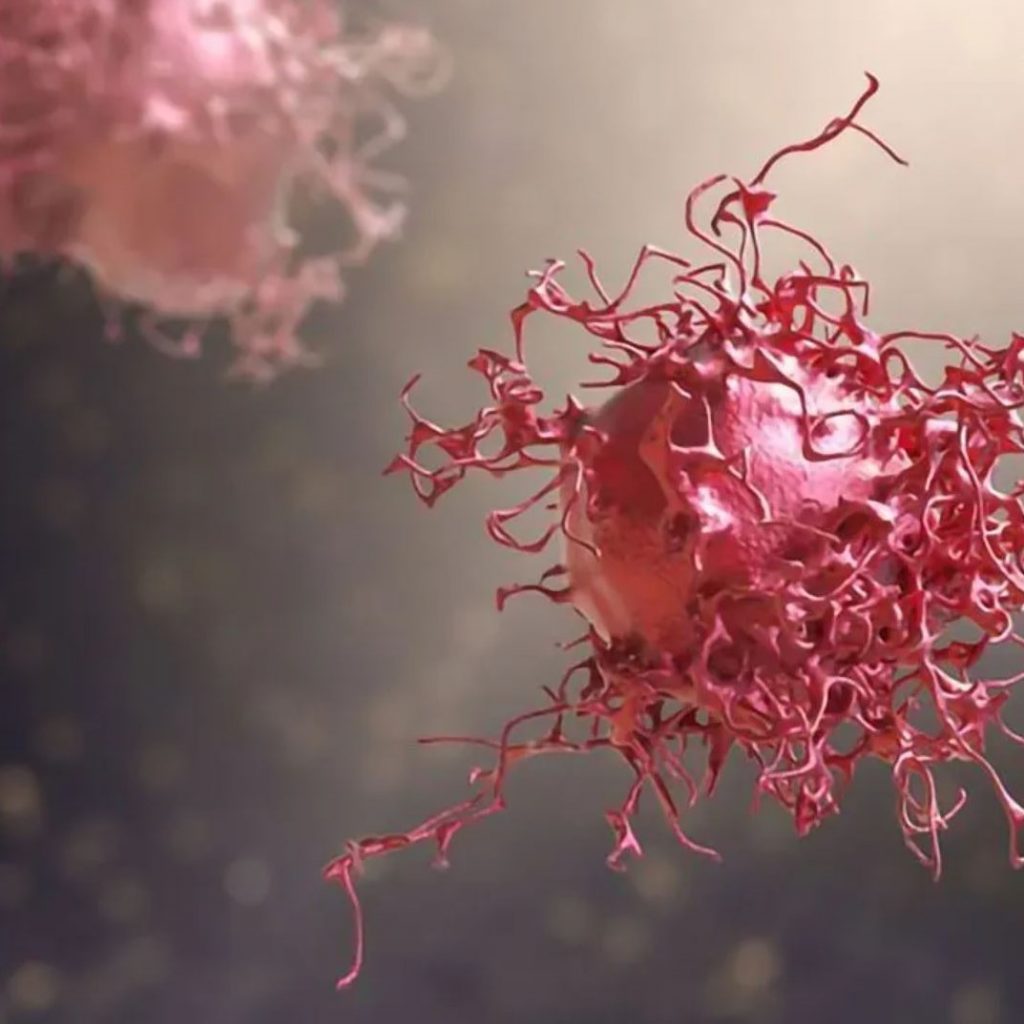
Related Press:
- “Studies Delve Deep into the Protein Machinery of Cancer Cells.” NCI (4 Nov 2021)
- “From COVID to cancer, gene-mapping tool could ‘revolutionize’ treatment“. SF Chronicle (2 Oct 2021)
- “Moonshot Project Aims to Understand and Beat Cancer Using Protein Maps“. Singularity Hub (5 Oct 2021)
- “Looking Beyond DNA to See Cancer with New Clarity,” features Trey Ideker, PhD.
- See also FierceBiotech, AXIOS, MSN, and Times of San Diego